Defect detection is one of the most common use cases of computer vision in manufacturing. Modern Visual AI technologies rely on powerful cloud-based servers that allow them to rapidly ingest visual information for machine-learning training purposes. By training a computer vision system with hundreds of thousands or millions of images of specific types of product defects, these systems can learn to rapidly identify similar defects with a high degree of accuracy. Visual AI defect detection systems can identify flaws like bottles missing bottlecaps, cracks in pipelines, poorly painted surfaces, missing parts, broken items, misshaped items, cracked glass, cracked metal casings, and virtually any other type of errors that human visual inspectors identify.
However, there are a number of other simpler use cases for computer vision systems that can generate higher Return on Investment (ROI) for manufacturing organizations with faster payback periods. We will explore a few of those use cases in this post.
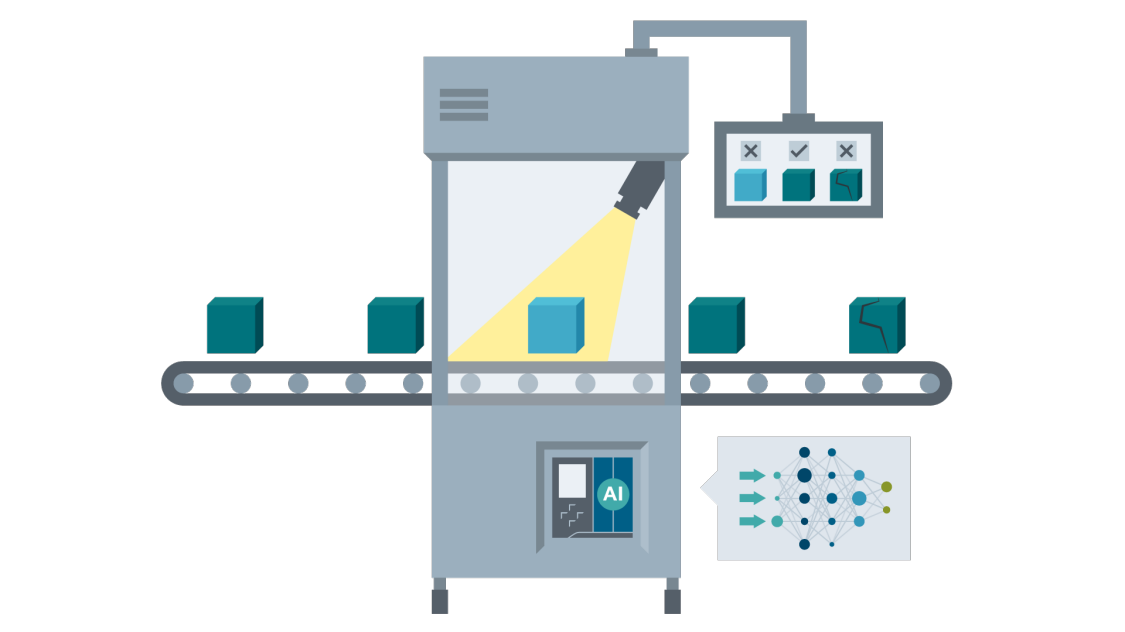